Controlling AI through compute may be necessary, but it won’t be easy.
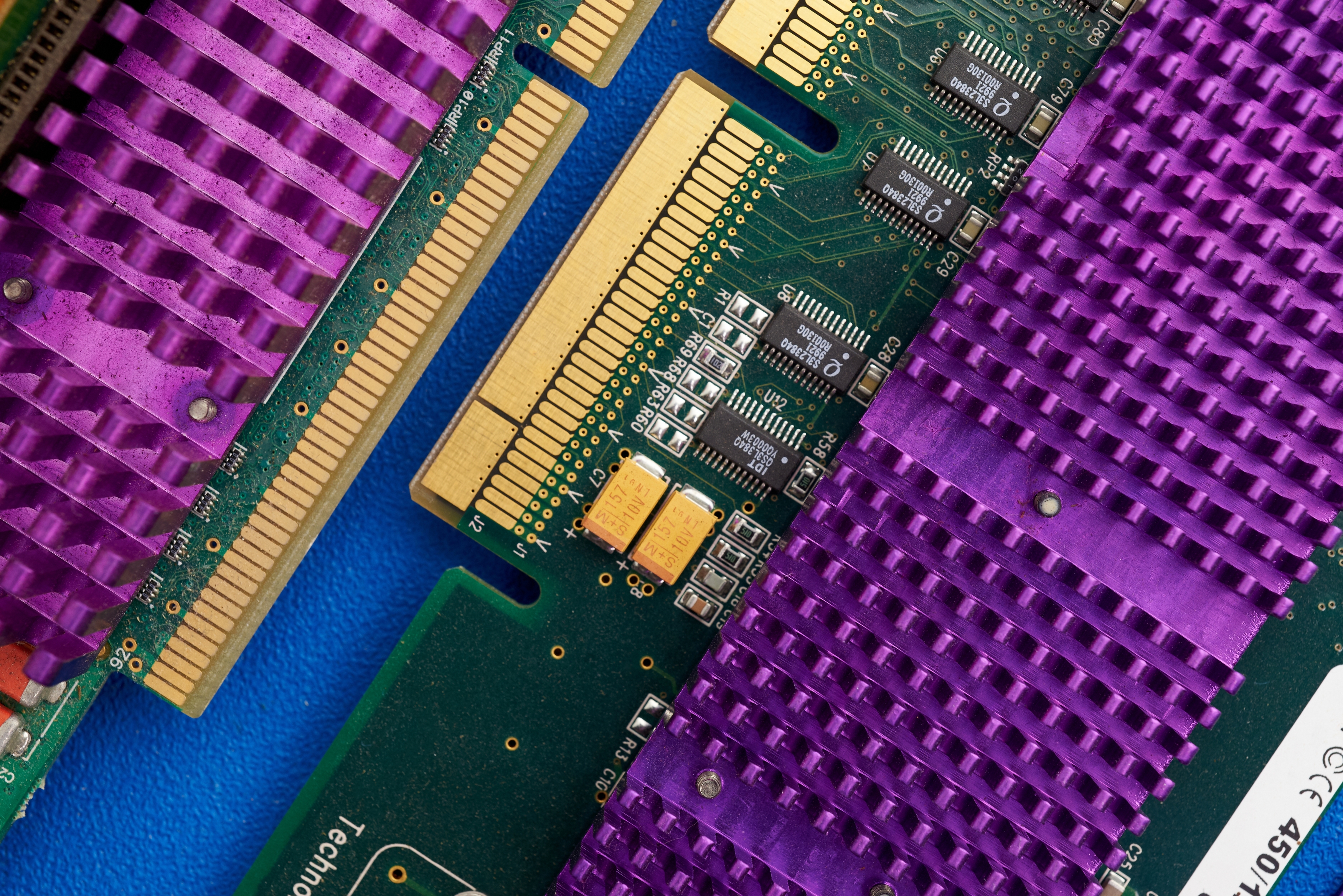
The continued development of artificial intelligence (AI) will consistently rely on the so-called AI triad: data, algorithms, and compute—the computing power AIs run on to train and deploy. Effective AI regulation requires that governments exercise some control over each part of this triad. Yet, while legislators and scholars have fervently debated the messy contours of regulating the triad’s first two legs (data and algorithms), they have put less emphasis on the third (compute)—that is, until now.
Because of compute’s central importance in driving AI growth, governments and regulators worldwide are beginning to turn their attention to the question of how it can be harnessed to shape the future of AI.
The idea of compute governance emerged from governments’ desire to secure AI supply chains, direct development within the industry, and set effective thresholds for private compliance with security-oriented policies. This article assesses the emergence of compute-based AI regulation, its benefits and drawbacks, and the accompanying legal challenges to effective implementation, at both national and international levels.
Domestic Compute Governance
Leading AI companies are rushing to acquire more compute because of its critical role in AI development. Much of the growth in AI capabilities over the past decade can be explained as a function of increases in the volume of compute that is deployed in AI training. Put simply, the more compute that developers use to train an AI model, the more advanced that model is likely to become.
As others have argued recently in Lawfare, compute is a powerful tool for governments to use toward a number of different policy ends. Governments may wish to track compute production and distribution—both within and across their borders—to gain greater visibility into the pace and direction of AI progress. Governments already use compute export controls, subsidies, and trade policies to control the speed of AI development and influence which companies and countries remain at the forefront.
Compute governance could also potentially be used to enforce an AI regulatory regime. A number of proposals have been made to harness the compute bottleneck in AI development and use chip governance to monitor compliance with forthcoming rules or regulations. Moreover, recent developments in chip technology open the possibility that firmware mechanisms could be implemented on AI chips themselves (“on-chip” mechanisms), which would allow regulators to robustly verify that chips are being trained to serve their AI developer’s purported purpose.
If the technology proves feasible, such a verification regime might first arise at a national level—as a means for governments to confidently know whether companies within their jurisdiction are complying with any domestic legal requirements around the training of AI models.
Both the EU and the U.S. have started to roll out compute-oriented regulations. The EU’s new AI Act introduces strict requirements on models that are deemed to pose “systemic risk,” calculated on the basis of the volume of compute used to power these models (this is currently set at 10^25 FLOPS, or floating-point operations per second, the standard measure for compute). Similarly, in the U.S., President Biden’s executive order on AI pulls several compute levers. It requires companies using models with 10^26 FLOPS capability to report plans for foundation model training runs and deployment to the federal government. It also requires providers of cloud computing services to adopt know-your-customer procedures to gain visibility over chip usage and determine whether foreign entities are accessing U.S. data centers to train AI models.
In the future, technology permitting, both the EU and the U.S. may even use on-chip mechanisms to verify a company’s compliance with model training regulations, a proposal previously floated by OpenAI’s Yonadav Shavit.
International Compute Governance
Given the global nature of the AI industry, comprehensive and effective compute governance inevitably means international compute governance.
Many countries have an interest in stemming the flow of chips to bad actors—criminal networks and terrorist groups—who might use them to train their own AI models or build custom versions of models that have already been released (a process known as fine-tuning). Bad actors with access to AI could potentially propagate complex cyberattacks or disinformation campaigns, or even reverse-engineer molecular compositions to design potent nerve agents “without much in the way of effort.” If the U.S. and its allies want to keep AI out of criminal or terrorist operations, it can’t just stem the flow to bad actors at home—it must coordinate with other countries to ensure adversarial non-state actors do not get compute from someone else.
Developing states with emerging tech sectors may also worry about getting left behind in the race for compute, and many of them have voiced an interest in coordinating compute-resources so that their tech sectors can still make meaningful progress—a concern that has been echoed in UN processes on AI governance. A report published by the UN’s AI Advisory Body, for example, expresses a desire for “federated access” to compute as a “a more lasting solution” to “ensuring AI is deployed for the common good.” In addition, the UN’s Global Digital Compact’s zero draft commits to “foster[ing] South-South and triangular cooperation around AI compute.”
Finally, international governance is needed because the supply chain for advanced semiconductors is the most complex industrial system in human history. It is spread throughout much of the world, implicating different geopolitical interests for different countries. Computer chips are made with rare earth metals such as scandium, titanium, and palladium, collectively mined in places as diverse as Germany, Italy, Japan, Kazakhstan, the Philippines, Russia, and South Africa. Midstream equipment for chip manufacturing such as wafers, photomasks, and “electronic gases” are concentrated in China, France, Germany, Japan, South Korea, and Taiwan. Open and consistent discussions about preserving access to the AI supply chain may serve a critical tension-reducing function in the future.
International coordination could take place either between a subset of states (such as the U.S. and its allies) or at a comprehensive global level. While the latter may seem less politically achievable, there have already been proposals—from industry, academia, and the UN secretary-general—for the establishment of a new international institution to monitor and verify compliance with any future rules on AI governance that states develop at a global level. Such a body could operate as an “IAEA for AI”—an international body with a dual mandate to distribute the benefits of AI technology globally and ensure that AI development is safe and aligned with principles agreed upon by states.
How would this body function? Just as the International Atomic Energy Agency (IAEA) monitors the use of fissile material in nuclear energy and weapons plants, a similar verification body could track the global flow of chips and verify that the compute deployed by companies to train advanced frontier AI models corroborates what AI developers say they’re using the compute for. Near-term technology, such as the on-chip mechanisms discussed above, could enable the relevant international body to confidently verify how actors are using compute. In turn, governments could tailor their interventions to those limited cases where an AI’s development might pose widespread risk.
The international community has already started to move in this direction. The Group of Seven (G7), Council of Europe, and other international forums are all pursuing regional and international AI governance strategies that indicate a willingness to coordinate on compute regulation in the coming years. The September UN Summit of the Future, facilitated by its UN High-Level Advisory Body on AI, serves as one core forum where states are expected to express their positions on access to compute power, and sign a “Global Digital Compact.”
Overcoming Domestic Challenges
In spite of its promises, however, harnessing compute governance to guide AI development raises significant legal, policy, and feasibility challenges—ranging from implementation obstacles in domestic and international jurisdictions to potential abuses of power by governments or regulators.
At the domestic level, privacy rights and norms will likely pose an obstacle to the enforcement of a compute governance regime. Unlike many other dual-use technologies that the U.S. military either helped invent or guided to market—consider the internet, avionics, and space systems—AI development is concentrated in the private sector. For context, of the 35 most significant machine learning breakthroughs in 2022, 32 were led by industry. While certain AI labs have experimented with nonprofit and other nontraditional corporate structures, almost all AI development today is being funded by private capital and driven by commercial interests. An effective compute governance regime must therefore carefully navigate this public-private tension, to ensure compliance by private-sector actors whose actions are motivated by a very different set of incentives than their governments.
Using the long arm of the law to verify how private entities are using chips poses a host of significant privacy and security challenges. In particular: Who might serve as “verifiers,” and how are they to be trusted? How will the flow of information they collect be kept secure? What exactly is that information, and does exposing it to government surveillance violate personal privacy rights or proprietary interests? These are all questions policymakers will need to have clear answers to in order to successfully implement a compute verification system.
As policymakers continue to debate these questions related to designing a compute regulatory regime, they will need to establish guardrails around the types of information collected. For example, on-chip governance mechanisms should be deployed to assess only whether AI developers are plausibly training their AIs for their stated purpose. This would mean that on-chip firmware should collect only a limited set of critical information, such as snapshots of the AI model weights or whether the training of the model surpasses a compute-usage threshold, not the data it is trained on itself.
Yet mandating chip firmware for the purposes of verifying compliance with regulation also raises corporate privacy concerns. Consider how a government monitor might implement an on-chip verification scheme. Apple, for example, currently uses an on-chip mechanism to prevent unauthorized applications from being installed on iPhones. Should the FBI or another government agency, leveraging its authority under this hypothetical compute governance regime, be permitted to access that mechanism and log the information it collects into a national database tracking chip use, access, and location? It is hard to imagine enthusiastic private-sector compliance, especially in light of the 2016 San Bernardino incident, where the FBI insisted Apple grant it access to a suspected terrorist’s iPhone. Apple wrote an impassioned brief challenging the FBI’s legal right to make such a demand and highlighting the grave risk enforcement would pose to consumer and industry privacy. But before the dispute was heard in district court, the FBI retained a private actor to break into the phone at issue, leaving in limbo the legal question of whether a government action can require a private company to comply with such a regulation.
Future chip-governance policymakers and enforcers would be wise to take a lesson from this incident. For instance, policymakers should expect some corporate pushback to implementing an on-chip verification system, even if the core information collected does not reveal private information. This is because, at least in the eyes of traditional chip users, sharing information—even with secure government monitors or even for national security purposes—can still disturb the integrity of their safety ecosystem.
At the same time, the largest AI labs in the U.S.—those likely with the greatest exposure to a national compute governance framework—have already signaled their willingness to work with the government on AI safety and security issues, especially regarding efforts to keep compute out of the hands of bad actors. Most notably, in a Senate hearing, OpenAI CEO Sam Altman explicitly called for the U.S. to lead the creation of an international compute verification agency.
The positive attitude many AI labs have toward government collaboration is further borne out by the fact that they have signed onto the Biden-Harris administration’s voluntary commitments on AI safety, promising to report vulnerabilities in their systems as well as updates on their models’ “capabilities, limitations, and areas of appropriate and inappropriate use.” The administration expressed its intention that these voluntary commitments will contribute more broadly to developing a “strong international framework to govern the development and use of AI.” Given the willingness of AI labs to share security-critical information with government entities to date, implementing firmware on chips may be a lower-hanging fruit than privacy critics expect.
Overcoming International Challenges
Though the willingness of industry-leading American companies to submit to compute regulation is encouraging, for a full-fledged international regime to be effective, the endorsement of foreign corporations and governments would also be required.
Securing these endorsements would be no small task, especially in light of some would-be partners taking issue with Biden’s AI executive order. France’s AI Commission, for instance, recently released a statement responding to the executive order, asserting that its provisions “contribute to reinforcing American dominance by slowing the development of foreign AI capabilities”—suggesting that the coming years might well be characterized by AI protectionism rather than intergovernmental cooperation. Indeed, conflicting values among America and its closest allies has proved to be an enduring source of tension in other transatlantic tech governance contexts (for example, between the U.S. and EU on data sharing and user privacy). States relatively new to the AI scene, such as France, Saudi Arabia, South Korea, Singapore, and the United Arab Emirates, are also beginning to develop their own AI industries and may be reluctant to sign on to a U.S.-led global regime that would restrict their ability to develop a domestic AI sector. This is not to mention the inherent difficulty in getting U.S. strategic rivals such as China to join an international compute governance mechanism.
Given the pace of AI development, and the threats that come with it, the amount of time needed to establish an international governance regime poses yet another challenge. It was seven long years between the initial U.S. proposal for international control of nuclear material (the Acheson-Lilienthal & Baruch Plans of 1946) and President Eisenhower’s “Atoms for Peace” speech that kicked off international discussions in earnest. The IAEA Statute then took a further three years to negotiate and another year to come into force. Given the pace of AI technical developments, governments simply do not have the same time.
Nevertheless, momentum is certainly growing for international coordination on AI, especially around potential safety concerns. The G7 is forging ahead on its process of coordinating its members’ AI regimes (the Hiroshima Process), and the U.S. government has recently indicated its willingness to work with China and other governments in tackling the greatest potential risks from AI.
Conclusion
As governments around the world brace for an uncertain future of AI developments, compute governance, and specifically compute-based enforcement, should be a prominent component of AI regulatory regimes. Its booming AI industry and current absence of regulation leaves the U.S. well positioned to take the lead, either by developing its nascent domestic compute governance regulation or, in the spirit of Eisenhower, by spearheading the design of an international compute monitoring verification body. Though either pursuit would face formidable obstacles, legislators should take some comfort in knowing that the main constraint on regulatory innovation is political, not technological, feasibility.
– Diane Bernabei, James Baker, Cosimo L. Fabrizio, Published courtesy of Lawfare.