If you blinked, you may have missed a recent wave of global AI commitments, a notable consensus in the chilliest international climate since the Cold War.
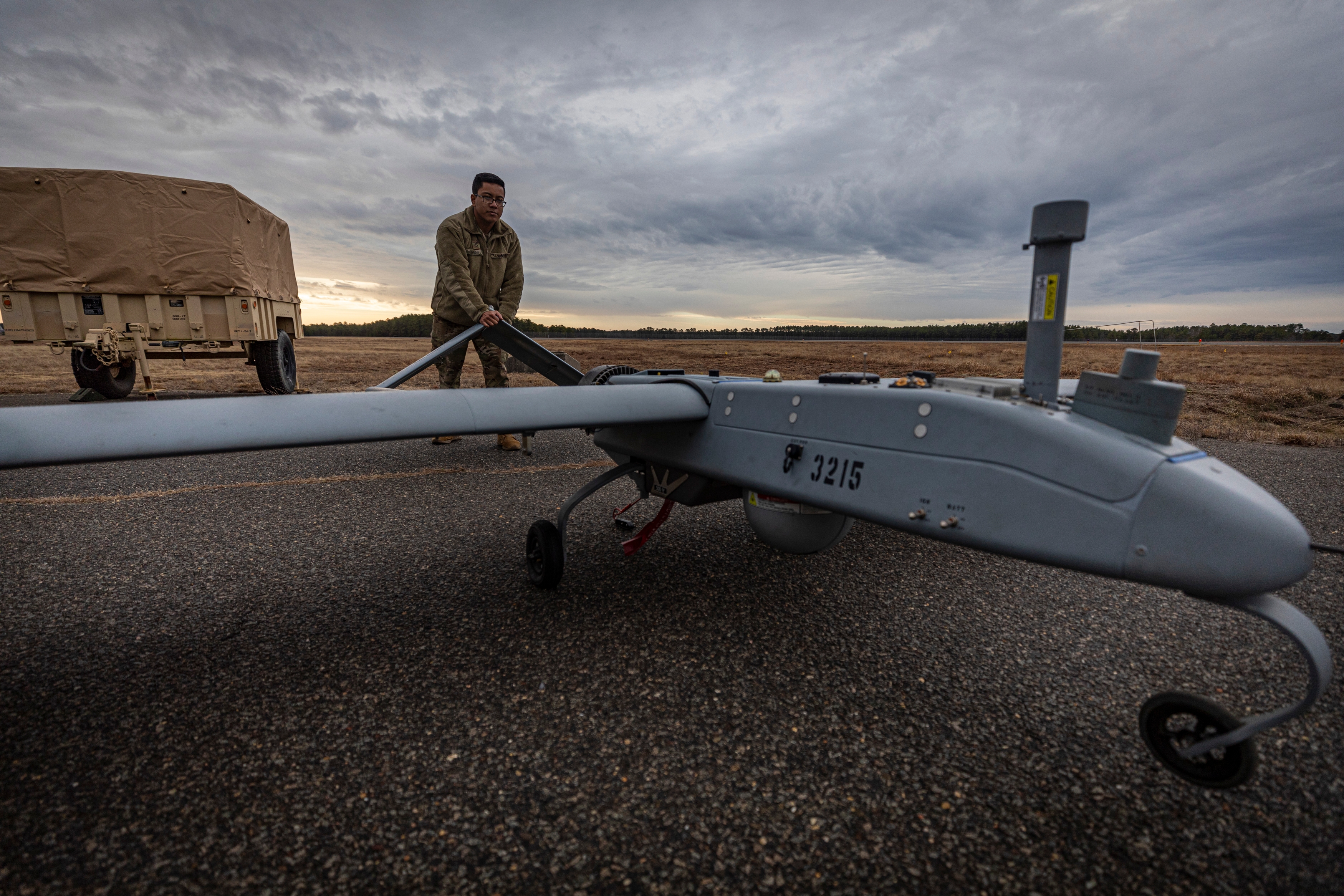
Despite rising geopolitical tensions, where adversarial nations find little to agree on, something of a global consensus seems to be taking shape around at least one issue: the use of artificial intelligence (AI) for defense. Efforts to further strengthen this regime will come to the fore this week in Washington, D.C., with the first meeting of signatories to the Political Declaration on Responsible Military Use of Artificial Intelligence and Autonomy this week (March 19-20).
This meeting comes hard on the heels of a series of AI governance efforts across 2023, including the February release of the U.S. Political Declaration on Responsible Military Use of Artificial Intelligence and Autonomy, a Chinese response with its own alternative Global AI Initiative proposal in October, and Vice President Harris’s November announcement of multilateral engagement with the Political Declaration. Finally, in late November, President Biden and Chinese President Xi Jinping met on the sidelines of the Asia-Pacific Economic Cooperation Summit, and while both sides tried to moderate expectations, the conversation nevertheless resulted in the reopening of military-to-military talks and an agreement to introduce an intergovernmental dialogue on AI.
Experts from private industry, the academy, and governments appear to agree that confidence-building measures (CBMs) offer an important step in the broader technology governance conversation. Popularized during the Cold War, CBMs are actions that reduce hostility, prevent conflict escalation, and improve trust between parties. Confidence-building measures have reemerged as an international governance method for a time when treaties and bans are increasingly politically difficult to create or even maintain. The reluctance to engage in formal, binding agreements can be tracked across areas of technology governance, from the numerous withdrawals from nuclear arms control treaties to the failed campaigns to ban lethal autonomous weapon systems. Against this backdrop, governments around the world are increasingly looking to mechanisms that are nonbinding and, in the U.S. case, do not require congressional approval. Indeed, CBMs have a low trust barrier to entry, no legal reinforcement, and can be revoked to protest competitor behavior.
The flexibility of CBMs makes them a key instrument for navigating the rapid changes in the AI landscape. Because states do not have perfect information about the capabilities or intentions of their allies and adversaries, formal and informal rules and exchanges can establish predictability around state behavior. But it’s harder to identify which CBMs are equally responsive to the technical realities imposed by AI technologies and the political requirements of our current moment.
Despite their lack of legal infrastructure and frequently narrow scope, CBMs can stabilize tense, unpredictable situations. A country willing to “build confidence” tends to be reassuring, as does a state continuing to follow CBMs in times of tension. For example, despite the heightened concern over possible nuclear escalation during the Russian invasion of Ukraine, the U.S. government could at least point to the Russian military’s continued sharing of information about missile launches through the National and Nuclear Risk Reduction Centers—a data exchange agreement originating in the Cold War.
An analogous approach to the data exchanges of the past will help societies prepare for the uncertainties posed by tomorrow’s AI models. A data exchange, in the context of military AI, involves sharing information—through red teaming and model evaluations, as explained below—to help parties understand the risks associated with AI models and even to steer models toward stabilizing behavior. Sharing this information would improve international transparency, which has long been regarded by international security practitioners as a stabilizing force on interstate relations.
Today’s frontier AI technologies—the name given to the next generation of large language models—are mostly developed in the private sector by a group of players sometimes referred to as “the frontier labs.” While frontier AI is not the only AI technology that is of concern to policymakers, it’s fair to describe these models as the catalyst that initiated a global wave of policymaker interest. Historically, confidence-building measures were the wheelhouse of militaries and defense ministries. Many examples of effective CBMs come from the Cold War and were exclusively governmental orchestrations. Today’s CBMs for the use of military AI will require a reimagining of the participants invited to the table—including frontier labs, academics, and civil society. As the field of AI continues to advance outside of government, it is well worth examining how innovators can play a role in identifying, communicating, and reducing international risks surrounding AI.
Further Fractures in the International Order?
Large language models can take on a range of military- or defense-related tasks that once would have required access to models developed for a specific purpose. These tasks include intelligence data summarization, target identification and selection, decision support, labeling geospatial imagery, automating cyber capabilities, and even integration into nuclear command and control. Today’s foundation models are general purpose, meaning that a single base model can be fine-tuned for seemingly dissimilar use cases across all industries.
AI’s general-purpose character complicates the core problem that plagues adversarial relationships: How do parties anticipate, distinguish, and interpret the actions of other states under imperfect information, especially when possession of the technology cannot predict how a party intends to use it? In practice, international stability depends on understanding the differences between offensive and defensive behavior, accidental and intentional escalation, and malicious versus civilian applications of nuclear, chemical, and biological sciences. These distinctions are traditionally maintained by establishing clear rules of the road on state behavior, whether through international law like the Geneva Conventions or through confidence-building measures like regular doctrine exchanges. However, it’s easier to lie if the technology provides scant evidence of planned wrongdoing, and easier still if there are no rules of the road that provide the language for legal retaliation or “naming and shaming.”
In addition to its general-purpose character, AI poses risks to international stability because it’s a strategically ambiguous technology, meaning it’s not clear whether the use of AI in a live conflict theater would trigger an escalatory spiral. Just as problematically, it’s not clear if parties will use military AI to remain under an escalation threshold while simultaneously increasing the destructiveness and duration of the conflict. Evidence of the latter dynamic is already in motion; the reported deployments of autonomous weapons systems in Ukraine don’t appear to further trigger escalatory retaliations or spirals in comparison to remotely piloted drones, despite warnings that autonomous drone use in Ukraine is “reshaping” the future of war. More challenging is the fact that autonomy is a spectrum lacking a consensus definition, and so it would be difficult to know whether autonomous drones result in a qualitatively different reaction from an adversary, especially when drone strikes are already often followed by retaliation. The definition of autonomy in military contexts could be the focus of early confidence-building measures, alongside doctrinal and cultural exchanges.
AI models further risk international stability because they’re accessible. As technologies designed with the everyday consumer in mind, they are easy to use and are intended for widespread adoption. The proliferation of large language models can be constrained, though not stopped, through export controls on computational hardware. Models like Stable Diffusion can now run on mobile devices, while some AI models are already being combined with robotics. In China, the wide availability of language models by various companies has been dubbed the “war of a 100 models.” Accessibility is overwhelmingly beneficial to the general public, allowing greater entry into an “innovator class” that has historically been the purview of a small and highly-skilled elite workforce. But as any scholar of non-proliferation knows, widespread access can also empower a minority of actors to misuse the technologies and further risk international instability.
New Technologies Need New Safety Tools: Red Teaming and Evaluations
States are highly unlikely to commit to a new code of conduct without reference to a scientific baseline. Understanding AI risks requires access to empirical testing. Otherwise, parties could bluff, exaggerate, or under-exaggerate their AI capabilities. Enter red teaming and evaluations.
Red teaming and evaluations are distinct, though often complementary activities. Not to be confused with adversarial testing in cyber systems, which focuses on characterizing threat actors by type and capability and is concerned primarily with technical vulnerabilities, the red teaming of large language models is an exercise intended to surface capabilities that have social, political, and economic impacts. Its similarity to red teaming cyber systems stems from the fact that AI red teamers are often instructed to “think like a bad actor” and use the model with the intention of causing harm. By running simulations and attack scenarios, red teams help to identify novel capabilities, limitations and biases, and areas for improvement in foundation models. Red teaming is among the first steps toward identifying post-training mitigations, like safety classifiers that refuse harmful model requests, and allows developers to steer the model toward less risky behaviors.
Evaluations quantify model capabilities and model safety in various domains, including text analysis, legal knowledge, and the detection of security flaws in software code. As of the time of writing, evaluations remain an early-stage methodology and are still in development, though they often involve multiple-choice question and answers, simulations and puzzles, and A/B experiments. Many evaluations that set the standard of today, like the Massive Multitask Language Understanding (MMLU), are also open source and freely available—in part due to advancements in this space being driven primarily by researchers in academia and adopted by industry. In addition to their prospective usefulness for security goals, these techniques address many other kinds of issues (for example, social bias, alignment with user intent, safety for individual users) and are widely recognized as best practices. In military contexts, the tracking of model performance would allow the military to better understand the capability of various models—particularly as their AI systems change and develop over time. System cards also allow for the communication and disclosure of known vulnerabilities, decreasing the danger of inadvertent escalation created by the use of model-based systems. Should a disclosed vulnerability be at the root of an incident, statements that that incident was caused by a malfunction of the system, rather than its intended use, may be more credible—driving down the risk of inadvertent escalation. Vulnerability disclosures would also increase public accountability and strengthen the demand-side signal for further international cooperative efforts to drive down the risks posed by military-AI integration.
While red teams and evaluations are necessary, these exercises come with an important disclaimer: They offer only a partial view of a model’s capabilities. Both red teaming and evaluations are methods that depend on viewing a model’s behavior when prompted by a human. Frontier models are massive—containing trillions of parameters—and human red teamers and evaluators cannot spend enough time prompting or questioning the model to reveal the full scope of its capabilities. Yet, to date, red teaming and evaluations are likely the best tools available for preemptively understanding the societal risks posed by black-boxed models before they are deployed in the real world. More work is surely needed among AI firms (whether via institutions like the Frontier Model Forum or more informal mechanisms) to collaboratively develop the red teaming tools and methods for use in private industry and that might ultimately travel to government and military contexts.
Governments are also improving their capacity to red-team models and conduct evaluations of their own. Much of this capacity is housed within newly created national institutions, specifically within the National Institute of Standards and Technology’s (NIST’s) AI Safety Institute in the United States (USAISI) and its British counterpart, the United Kingdom AI Safety Institute (UKAISI). Less discussed is the potential of red teams and evaluations as useful tools for promoting international stability, cooperation, and social resilience. Specifically, they can have a role in equalizing the playing field between countries that have large military research and development ecosystems and those countries that do not but will nevertheless be affected by the changing security landscape. Such assessments would not only, for example, test a model’s applicability in domains like fusing and analyzing satellite data but also offer deeper insight into its safety and reliability in high-stakes environments.
Realistically, these tools require resources far beyond the recent $10 million suggested to the U.S. Senate Appropriations Committee. Thin funding places NIST’s AI safety agenda on life support, translating into trouble hiring machine learning talent in an industry that can offer salaries and compensation packages totaling in the high six figures and without the infamous timelines that characterize the government hiring process. By contrast, the U.K. government awarded the UKAISI 100 million pounds in funding. Even the UKAISI, however, limits misuse prevention to non-state actors based on their possible acquisition of unconventional weapons.
The AISIs do not—and should not be expected to—set the behavioral norms that guide state-to-state military interactions. However, their work provides a useful template for understanding how models are evaluated and the way experts think through safety evaluations. AISI capacity, though nascent, can be leveraged to support the recent U.S. Political Declaration on Responsible Military Use of Artificial Intelligence and Autonomy by exchanging red team insights and the results of evaluations between the declaration’s signatories. A collaboration between the U.S. Department of Defense and NIST would not be unprecedented, with both departments having collaborated in the past to build a unified cybersecurity framework.
Even allies are sometimes reluctant to exchange information that may reveal the architecture underlying a military capability. However, red teams and evaluations can improve transparency about a model’s capabilities with a reduced likelihood of revealing information about the methods used to build the model. As it applies to red teaming, at least, the transparency and security trade-off appears to have widespread buy-in. Notably, several AI labs partnered with the White House to host a public red teaming exercise in 2023 at DefCon, which allowed convention attendees to red team frontier lab models across a range of domains, including cyber. These events focus on improving public transparency about a model’s outputs rather than improving transparency into the model’s underlying architecture. Red teams could also be conducted privately as bilateral or multilateral exercises, similar to the diplomatic war games that are conducted today, and would allow participants to directly observe the model’s behavior in a controlled setting. If trust were sufficiently established, then parties could eventually exchange data set questionnaires and red team prompts (inputs designed by the human red teamer).
Building Consensus on the Shape of AI Risks
Confidence-building measures are either additive, where states choose to incorporate new protocols or behaviors, or restrictive, where states choose to limit behaviors or capabilities. Though the voluntary and adaptable nature of CBMs can encourage participation in a low-trust international environment, states will participate in a CBMs regime only if they share the same interpretation of the risk landscape. Given the ongoing contentious debate surrounding topics like existential risks and AI ethics, this should not be mistaken as self-evident. This norms misalignment could make the road to CBMs adoption a difficult one, as illustrated by the difficulty of engaging China in military-to-military communications on challenges that should, at first glance, be in the interest of all parties to resolve. Sharing red team and evaluation results, however, can provide the necessary empirical evidence to build a community of consensus, whether that consensus serves to confirm the existence of or deflate the hype surrounding AI risk.
Efforts to craft CBMs in the AI space are complicated by the role of non-state entities in the production of what is ultimately a dual-use technology. As such, engagement with nontraditional nongovernmental partners from around the world is essential for the success of the CBMs design process. The inclusion of these diverse partners, in combination with red teaming and evaluations, is an integral step toward establishing a community of states equipped with the knowledge to confront tomorrow’s AI threats and opportunities.
– Sarah Shoker, Andrew Reddie, Alan Hickey, Leah Walker, Published courtesy of Lawfare.